2024
Poya, Roman; Ortigosa, Rogelio; Gil, Antonio J.
Generalised tangent stabilised nonlinear elasticity: A powerful framework for controlling material and geometric instabilities Journal Article Forthcoming
In: International Journal for Numerical Methods in Engineering, Forthcoming.
@article{Poya2024,
title = {Generalised tangent stabilised nonlinear elasticity: A powerful framework for controlling material and geometric instabilities},
author = {Roman Poya and Rogelio Ortigosa and Antonio J. Gil},
year = {2024},
date = {2024-04-19},
urldate = {2024-04-19},
journal = {International Journal for Numerical Methods in Engineering},
keywords = {},
pubstate = {forthcoming},
tppubtype = {article}
}
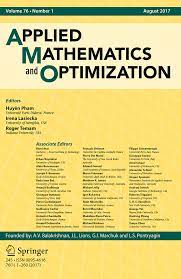
Ortigosa, Rogelio; Martínez-Frutos, Jesús; Mora-Corral, Carlos; Pedregal, Pablo; Periago, Francisco
Shape-programming in hyperelasticity through differential growth Journal Article
In: Applied Mathematics and Optimization, vol. 89, no. 49, 2024, ISSN: 1432-0606.
@article{Ortigosa2024b,
title = {Shape-programming in hyperelasticity through differential growth},
author = {Rogelio Ortigosa and Jesús Martínez-Frutos and Carlos Mora-Corral and Pablo Pedregal and Francisco Periago},
editor = {Springer},
url = {https://link.springer.com/10.1007/s00245-024-10117-6?utm_source=rct_congratemailt&utm_medium=email&utm_campaign=oa_20240323&utm_content=10.1007/s00245-024-10117-6},
doi = {10.1007/s00245-024-10117-6},
issn = {1432-0606},
year = {2024},
date = {2024-03-23},
urldate = {2024-12-01},
journal = {Applied Mathematics and Optimization},
volume = {89},
number = {49},
abstract = {This paper is concerned with the growth-driven shape-programming problem, which involves determining a growth tensor that can produce a deformation on a hyperelastic body reaching a given target shape. We consider the two cases of globally compatible growth, where the growth tensor is a deformation gradient over the undeformed domain, and the incompatible one, which discards such hypothesis. We formulate the problem within the framework of optimal control theory in hyperelasticity. The Hausdorff distance is used to quantify dissimilarities between shapes; the complexity of the actuation is incorporated in the cost functional as well. Boundary conditions and external loads are allowed in the state law, thus extending previous works where the stress-free hypothesis turns out to be essential. A rigorous mathematical analysis is then carried out to prove the well-posedness of the problem. The numerical approximation is performed using gradient-based optimisation algorithms. Our main goal in this part is to show the possibility to apply inverse techniques for the numerical approximation of this problem, which allows us to address more generic situations than those covered by analytical approaches. Several numerical experiments for beam-like and shell-type geometries illustrate the performance of the proposed numerical scheme.},
keywords = {},
pubstate = {published},
tppubtype = {article}
}
This paper is concerned with the growth-driven shape-programming problem, which involves determining a growth tensor that can produce a deformation on a hyperelastic body reaching a given target shape. We consider the two cases of globally compatible growth, where the growth tensor is a deformation gradient over the undeformed domain, and the incompatible one, which discards such hypothesis. We formulate the problem within the framework of optimal control theory in hyperelasticity. The Hausdorff distance is used to quantify dissimilarities between shapes; the complexity of the actuation is incorporated in the cost functional as well. Boundary conditions and external loads are allowed in the state law, thus extending previous works where the stress-free hypothesis turns out to be essential. A rigorous mathematical analysis is then carried out to prove the well-posedness of the problem. The numerical approximation is performed using gradient-based optimisation algorithms. Our main goal in this part is to show the possibility to apply inverse techniques for the numerical approximation of this problem, which allows us to address more generic situations than those covered by analytical approaches. Several numerical experiments for beam-like and shell-type geometries illustrate the performance of the proposed numerical scheme.
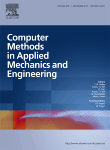
Klein, Dominik; Ortigosa, Rogelio; Martínez-Frutos, Jesús; Weeger, Oliver
Nonlinear electro-elastic finite element analysis with neural network constitutive models Journal Article
In: Computer Methods in Applied Mechanics and Engineering, vol. 425, 2024, ISBN: 1879-2138.
@article{Klein2024,
title = {Nonlinear electro-elastic finite element analysis with neural network constitutive models},
author = {Dominik Klein and Rogelio Ortigosa and Jesús Martínez-Frutos and Oliver Weeger},
editor = {Elsevier},
url = {https://www.sciencedirect.com/science/article/pii/S004578252400166X},
doi = {https://doi.org/10.1016/j.cma.2024.116910},
isbn = {1879-2138},
year = {2024},
date = {2024-03-15},
urldate = {2024-07-01},
journal = {Computer Methods in Applied Mechanics and Engineering},
volume = {425},
abstract = {In the present work, the applicability of physics-augmented neural network (PANN) constitutive models for complex electro-elastic finite element analysis is demonstrated. For the investigations, PANN models for electro-elastic material behavior at finite deformations are calibrated to different synthetically generated datasets describing the constitutive response of dielectric elastomers. These include an analytical isotropic potential, a homogenised rank-one laminate, and a homogenised metamaterial with a spherical inclusion. Subsequently, boundary value problems inspired by engineering applications of composite electro-elastic materials are considered. Scenarios with large electrically induced deformations and instabilities are particularly challenging and thus necessitate extensive investigations of the PANN constitutive models in the context of finite element analyses. First of all, an excellent prediction quality of the model is required for very general load cases occurring in the simulation. Furthermore, simulation of large deformations and instabilities poses challenges on the stability of the numerical solver, which is closely related to the constitutive model. In all cases studied, the PANN models yield excellent prediction qualities and a stable numerical behavior even in highly nonlinear scenarios. This can be traced back to the PANN models excellent performance in learning both the first and second derivatives of the ground truth electro-elastic potentials, even though it is only calibrated on the first derivatives. Overall, this work demonstrates the applicability of PANN constitutive models for the efficient and robust simulation of engineering applications of composite electro-elastic materials.
},
keywords = {},
pubstate = {published},
tppubtype = {article}
}
In the present work, the applicability of physics-augmented neural network (PANN) constitutive models for complex electro-elastic finite element analysis is demonstrated. For the investigations, PANN models for electro-elastic material behavior at finite deformations are calibrated to different synthetically generated datasets describing the constitutive response of dielectric elastomers. These include an analytical isotropic potential, a homogenised rank-one laminate, and a homogenised metamaterial with a spherical inclusion. Subsequently, boundary value problems inspired by engineering applications of composite electro-elastic materials are considered. Scenarios with large electrically induced deformations and instabilities are particularly challenging and thus necessitate extensive investigations of the PANN constitutive models in the context of finite element analyses. First of all, an excellent prediction quality of the model is required for very general load cases occurring in the simulation. Furthermore, simulation of large deformations and instabilities poses challenges on the stability of the numerical solver, which is closely related to the constitutive model. In all cases studied, the PANN models yield excellent prediction qualities and a stable numerical behavior even in highly nonlinear scenarios. This can be traced back to the PANN models excellent performance in learning both the first and second derivatives of the ground truth electro-elastic potentials, even though it is only calibrated on the first derivatives. Overall, this work demonstrates the applicability of PANN constitutive models for the efficient and robust simulation of engineering applications of composite electro-elastic materials.
Klein, Dominik; Ortigosa, Rogelio; Martínez-Frutos, Jesús; Weeger, Oliver
Neural networks meet hyperelasticity: On limits of polyconvexity Journal Article Forthcoming
In: Journal of the Mechanics and Physics of Solids, Forthcoming.
@article{Klein2024b,
title = {Neural networks meet hyperelasticity: On limits of polyconvexity},
author = {Dominik Klein and Rogelio Ortigosa and Jesús Martínez-Frutos and Oliver Weeger},
editor = {Elsevier},
year = {2024},
date = {2024-03-15},
urldate = {2024-03-15},
journal = {Journal of the Mechanics and Physics of Solids},
keywords = {},
pubstate = {forthcoming},
tppubtype = {article}
}
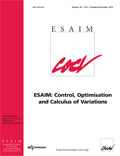
Ortigosa, Rogelio; Martinez-Frutos, Jesus; Periago, Francisco
Probability-of-failure-based optimization for Random pdes through concentration-of-measure Inequalities Journal Article Forthcoming
In: ESAIM: Control, Optimisation and Calculus of Variations, Forthcoming.
@article{Ortigosa2024,
title = {Probability-of-failure-based optimization for Random pdes through concentration-of-measure Inequalities},
author = {Rogelio Ortigosa and Jesus Martinez-Frutos and Francisco Periago},
doi = {doi.org/10.1051/cocv/2023075},
year = {2024},
date = {2024-03-01},
urldate = {2024-03-01},
journal = {ESAIM: Control, Optimisation and Calculus of Variations},
abstract = {Control and optimization problems constrained by partial differential equations (PDEs)
with random input data and that incorporate probabilities of failure in their formulations are numerically
extremely challenging, since the computational cost of estimating the tails of a probability
distribution is prohibitive in many situations encountered in real-life engineering problems. In addition,
probabilities of failure are often discontinuous and include huge flat regions where gradients vanish.
Based on the McDiarmid concentration-of-measure inequality, this paper proposes a new functional
which provides a tight and smooth bound for the probability of a given random functional of exceeding
a prescribed threshold parameter. Hence, this approach relieves the above-mentioned difficulties in
the case where the solution map is convex with respect to the random parameter, as in the case of
a deterministic differential operator and the random parameter appearing linearly in the right-hand
side term. Well-posedness of the corresponding optimal control problem is established and the viability
of the proposed method is numerically illustrated by two benchmarks examples arising in topology
optimization and optimal control theory.},
keywords = {},
pubstate = {forthcoming},
tppubtype = {article}
}
Control and optimization problems constrained by partial differential equations (PDEs)
with random input data and that incorporate probabilities of failure in their formulations are numerically
extremely challenging, since the computational cost of estimating the tails of a probability
distribution is prohibitive in many situations encountered in real-life engineering problems. In addition,
probabilities of failure are often discontinuous and include huge flat regions where gradients vanish.
Based on the McDiarmid concentration-of-measure inequality, this paper proposes a new functional
which provides a tight and smooth bound for the probability of a given random functional of exceeding
a prescribed threshold parameter. Hence, this approach relieves the above-mentioned difficulties in
the case where the solution map is convex with respect to the random parameter, as in the case of
a deterministic differential operator and the random parameter appearing linearly in the right-hand
side term. Well-posedness of the corresponding optimal control problem is established and the viability
of the proposed method is numerically illustrated by two benchmarks examples arising in topology
optimization and optimal control theory.

Pérez-Escolar, Alberto; Martinez-Frutos, Jesus; Ortigosa, Rogelio; Ellmer, Nathan; Gil, Antonio J.
Learning nonlinear constitutive models in finite strain electromechanics with Gaussian process predictors Journal Article
In: Computational Mechanics, 2024, ISBN: 1432-0924.
@article{Pérez-Escolar2024,
title = {Learning nonlinear constitutive models in finite strain electromechanics with Gaussian process predictors},
author = {Alberto Pérez-Escolar and Jesus Martinez-Frutos and Rogelio Ortigosa and Nathan Ellmer and Antonio J. Gil},
editor = {Springer},
doi = {10.1007/s00466-024-02446-8},
isbn = {1432-0924},
year = {2024},
date = {2024-02-20},
urldate = {2024-03-01},
journal = {Computational Mechanics},
abstract = {This paper introduces a metamodelling technique that employs gradient-enhanced Gaussian Process Regression (GPR) to emulate diverse internal energy densities based on the deformation gradient tensor F and electric displacement eld D0. The approach integrates principal invariants as inputs for the surrogate internal energy density, enforcing physical constraints like material frame indi erence and symmetry. This technique enables accurate interpolation of energy and its derivatives, including the rst Piola-Kirchho stress tensor and material electric field. The method ensures stress and electric eld-free conditions at the origin, which is challenging with regression-based methods like neural networks. The paper highlights that using invariants of the dual potential of internal energy density, i.e., the free energy density dependent on the material electric eld E0, is inappropriate. The saddle point nature of the latter contrasts with the convexity of the internal energy density, creating challenges for GPR or Gradient Enhanced GPR models using invariants of F and E0 (free energy-based GPR), compared to those involving F and D0 (internal energy-based GPR). Numerical examples within a 3D Finite Element framework assess surrogate
model accuracy across challenging scenarios, comparing displacement and stress elds with ground-truth analytical models. Cases include extreme twisting and electrically induced wrinkles, demonstrating practical applicability and robustness of the proposed approach.},
keywords = {},
pubstate = {published},
tppubtype = {article}
}
This paper introduces a metamodelling technique that employs gradient-enhanced Gaussian Process Regression (GPR) to emulate diverse internal energy densities based on the deformation gradient tensor F and electric displacement eld D0. The approach integrates principal invariants as inputs for the surrogate internal energy density, enforcing physical constraints like material frame indi erence and symmetry. This technique enables accurate interpolation of energy and its derivatives, including the rst Piola-Kirchho stress tensor and material electric field. The method ensures stress and electric eld-free conditions at the origin, which is challenging with regression-based methods like neural networks. The paper highlights that using invariants of the dual potential of internal energy density, i.e., the free energy density dependent on the material electric eld E0, is inappropriate. The saddle point nature of the latter contrasts with the convexity of the internal energy density, creating challenges for GPR or Gradient Enhanced GPR models using invariants of F and E0 (free energy-based GPR), compared to those involving F and D0 (internal energy-based GPR). Numerical examples within a 3D Finite Element framework assess surrogate
model accuracy across challenging scenarios, comparing displacement and stress elds with ground-truth analytical models. Cases include extreme twisting and electrically induced wrinkles, demonstrating practical applicability and robustness of the proposed approach.
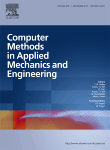
Ellmer, Nathan; Ortigosa, Rogelio; Martinez-Frutos, Jesus; Gil, Antonio J.
Gradient enhanced gaussian process regression for constitutive modelling in finite strain hyperelasticity Journal Article
In: Computer Methods in Applied Mechanics and Engineering, vol. 418, iss. PART B, pp. 116547, 2024, ISBN: 1879-2138.
@article{Ellmer2024,
title = {Gradient enhanced gaussian process regression for constitutive modelling in finite strain hyperelasticity},
author = {Nathan Ellmer and Rogelio Ortigosa and Jesus Martinez-Frutos and Antonio J. Gil},
editor = {Elsevier},
doi = {10.1016/j.cma.2023.116547},
isbn = {1879-2138},
year = {2024},
date = {2024-01-05},
urldate = {2024-01-05},
journal = {Computer Methods in Applied Mechanics and Engineering},
volume = {418},
issue = {PART B},
pages = {116547},
abstract = {This paper introduces a metamodelling technique that leverages gradient-enhanced Gaussian process regression (also known as gradient-enhanced Kriging), effectively emulating the response of diverse hyperelastic strain energy densities. The approach adopted incorporates principal invariants as inputs for the surrogate of the strain energy density. This integration enables the surrogate to inherently enforce fundamental physical constraints, such as material frame indifference and material symmetry, right from the outset. The proposed approach provides accurate interpolation for energy and the first Piola–Kirchhoff stress tensor (e.g. first order derivatives with respect to inputs). The paper presents three notable innovations. Firstly, it introduces the utilisation of Gradient-Enhanced Kriging to approximate a diverse range of phenomenological models, encompassing numerous isotropic hyperelastic strain energies and a transversely isotropic potential. Secondly, this study marks the inaugural application of this technique for approximating the effective response of composite materials. This includes rank-one laminates, for which analytical solutions are feasible. However, it also encompasses more complex composite materials characterised by a Representative Volume Element (RVE) comprising an elastomeric matrix with a centred spherical inclusion. This extension opens the door for future application of this technique to various RVE types, facilitating efficient three-dimensional computational analyses at the macro-scale of such composite materials, significantly reducing computational time compared to FEM. The third innovation, facilitated by the integration of these surrogate models into a 3D Finite Element computational framework, lies in the assessment of these models scenarios encompassing intricate cases of extreme twisting and more importantly, buckling instabilities in thin-walled structures, thereby highlighting both the practical applicability and robustness of the proposed approach.},
keywords = {},
pubstate = {published},
tppubtype = {article}
}
This paper introduces a metamodelling technique that leverages gradient-enhanced Gaussian process regression (also known as gradient-enhanced Kriging), effectively emulating the response of diverse hyperelastic strain energy densities. The approach adopted incorporates principal invariants as inputs for the surrogate of the strain energy density. This integration enables the surrogate to inherently enforce fundamental physical constraints, such as material frame indifference and material symmetry, right from the outset. The proposed approach provides accurate interpolation for energy and the first Piola–Kirchhoff stress tensor (e.g. first order derivatives with respect to inputs). The paper presents three notable innovations. Firstly, it introduces the utilisation of Gradient-Enhanced Kriging to approximate a diverse range of phenomenological models, encompassing numerous isotropic hyperelastic strain energies and a transversely isotropic potential. Secondly, this study marks the inaugural application of this technique for approximating the effective response of composite materials. This includes rank-one laminates, for which analytical solutions are feasible. However, it also encompasses more complex composite materials characterised by a Representative Volume Element (RVE) comprising an elastomeric matrix with a centred spherical inclusion. This extension opens the door for future application of this technique to various RVE types, facilitating efficient three-dimensional computational analyses at the macro-scale of such composite materials, significantly reducing computational time compared to FEM. The third innovation, facilitated by the integration of these surrogate models into a 3D Finite Element computational framework, lies in the assessment of these models scenarios encompassing intricate cases of extreme twisting and more importantly, buckling instabilities in thin-walled structures, thereby highlighting both the practical applicability and robustness of the proposed approach.