2024
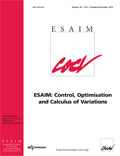
Ortigosa, Rogelio; Martinez-Frutos, Jesus; Periago, Francisco
Probability-of-failure-based optimization for Random pdes through concentration-of-measure Inequalities Journal Article
In: ESAIM: Control, Optimisation and Calculus of Variations, vol. 30, no. 66, 2024.
@article{Ortigosa2024,
title = {Probability-of-failure-based optimization for Random pdes through concentration-of-measure Inequalities},
author = {Rogelio Ortigosa and Jesus Martinez-Frutos and Francisco Periago},
doi = {https://doi.org/10.1051/cocv/2023075},
year = {2024},
date = {2024-09-23},
urldate = {2024-03-01},
journal = {ESAIM: Control, Optimisation and Calculus of Variations},
volume = {30},
number = {66},
abstract = {Control and optimization problems constrained by partial differential equations (PDEs)
with random input data and that incorporate probabilities of failure in their formulations are numerically
extremely challenging, since the computational cost of estimating the tails of a probability
distribution is prohibitive in many situations encountered in real-life engineering problems. In addition,
probabilities of failure are often discontinuous and include huge flat regions where gradients vanish.
Based on the McDiarmid concentration-of-measure inequality, this paper proposes a new functional
which provides a tight and smooth bound for the probability of a given random functional of exceeding
a prescribed threshold parameter. Hence, this approach relieves the above-mentioned difficulties in
the case where the solution map is convex with respect to the random parameter, as in the case of
a deterministic differential operator and the random parameter appearing linearly in the right-hand
side term. Well-posedness of the corresponding optimal control problem is established and the viability
of the proposed method is numerically illustrated by two benchmarks examples arising in topology
optimization and optimal control theory.},
keywords = {21996/PI/22},
pubstate = {published},
tppubtype = {article}
}
Control and optimization problems constrained by partial differential equations (PDEs)
with random input data and that incorporate probabilities of failure in their formulations are numerically
extremely challenging, since the computational cost of estimating the tails of a probability
distribution is prohibitive in many situations encountered in real-life engineering problems. In addition,
probabilities of failure are often discontinuous and include huge flat regions where gradients vanish.
Based on the McDiarmid concentration-of-measure inequality, this paper proposes a new functional
which provides a tight and smooth bound for the probability of a given random functional of exceeding
a prescribed threshold parameter. Hence, this approach relieves the above-mentioned difficulties in
the case where the solution map is convex with respect to the random parameter, as in the case of
a deterministic differential operator and the random parameter appearing linearly in the right-hand
side term. Well-posedness of the corresponding optimal control problem is established and the viability
of the proposed method is numerically illustrated by two benchmarks examples arising in topology
optimization and optimal control theory.
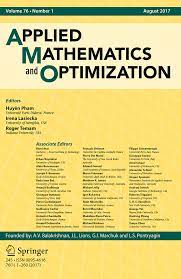
Ortigosa, Rogelio; Martínez-Frutos, Jesús; Mora-Corral, Carlos; Pedregal, Pablo; Periago, Francisco
Shape-programming in hyperelasticity through differential growth Journal Article
In: Applied Mathematics and Optimization, vol. 89, no. 49, 2024, ISSN: 1432-0606.
@article{Ortigosa2024b,
title = {Shape-programming in hyperelasticity through differential growth},
author = {Rogelio Ortigosa and Jesús Martínez-Frutos and Carlos Mora-Corral and Pablo Pedregal and Francisco Periago},
editor = {Springer},
url = {https://link.springer.com/10.1007/s00245-024-10117-6?utm_source=rct_congratemailt&utm_medium=email&utm_campaign=oa_20240323&utm_content=10.1007/s00245-024-10117-6},
doi = {10.1007/s00245-024-10117-6},
issn = {1432-0606},
year = {2024},
date = {2024-03-23},
urldate = {2024-12-01},
journal = {Applied Mathematics and Optimization},
volume = {89},
number = {49},
abstract = {This paper is concerned with the growth-driven shape-programming problem, which involves determining a growth tensor that can produce a deformation on a hyperelastic body reaching a given target shape. We consider the two cases of globally compatible growth, where the growth tensor is a deformation gradient over the undeformed domain, and the incompatible one, which discards such hypothesis. We formulate the problem within the framework of optimal control theory in hyperelasticity. The Hausdorff distance is used to quantify dissimilarities between shapes; the complexity of the actuation is incorporated in the cost functional as well. Boundary conditions and external loads are allowed in the state law, thus extending previous works where the stress-free hypothesis turns out to be essential. A rigorous mathematical analysis is then carried out to prove the well-posedness of the problem. The numerical approximation is performed using gradient-based optimisation algorithms. Our main goal in this part is to show the possibility to apply inverse techniques for the numerical approximation of this problem, which allows us to address more generic situations than those covered by analytical approaches. Several numerical experiments for beam-like and shell-type geometries illustrate the performance of the proposed numerical scheme.},
keywords = {21996/PI/22},
pubstate = {published},
tppubtype = {article}
}
This paper is concerned with the growth-driven shape-programming problem, which involves determining a growth tensor that can produce a deformation on a hyperelastic body reaching a given target shape. We consider the two cases of globally compatible growth, where the growth tensor is a deformation gradient over the undeformed domain, and the incompatible one, which discards such hypothesis. We formulate the problem within the framework of optimal control theory in hyperelasticity. The Hausdorff distance is used to quantify dissimilarities between shapes; the complexity of the actuation is incorporated in the cost functional as well. Boundary conditions and external loads are allowed in the state law, thus extending previous works where the stress-free hypothesis turns out to be essential. A rigorous mathematical analysis is then carried out to prove the well-posedness of the problem. The numerical approximation is performed using gradient-based optimisation algorithms. Our main goal in this part is to show the possibility to apply inverse techniques for the numerical approximation of this problem, which allows us to address more generic situations than those covered by analytical approaches. Several numerical experiments for beam-like and shell-type geometries illustrate the performance of the proposed numerical scheme.